Construction of a Prediction Model of the Shortest Annual Graduation by Machine Learning Using Learning Environment Data
Purchase or Subscription required for access
Purchase individual articles and papers
Subscribe for faster access!
Subscribe and receive access to 100,000+ documents, for only $19/month (or $150/year).
Already have access?
Individual Subscription
If you have an individual subscription, sign in here for access
Institutional Subscription
You don't appear to be accessing the site through a subscribing institution (your IP address is 3.149.231.55).
If your university, college, or library subscribes to LearnTechLib, you may be able access full text articles through a login page.
You can search for your instition by name or by location.
Authors
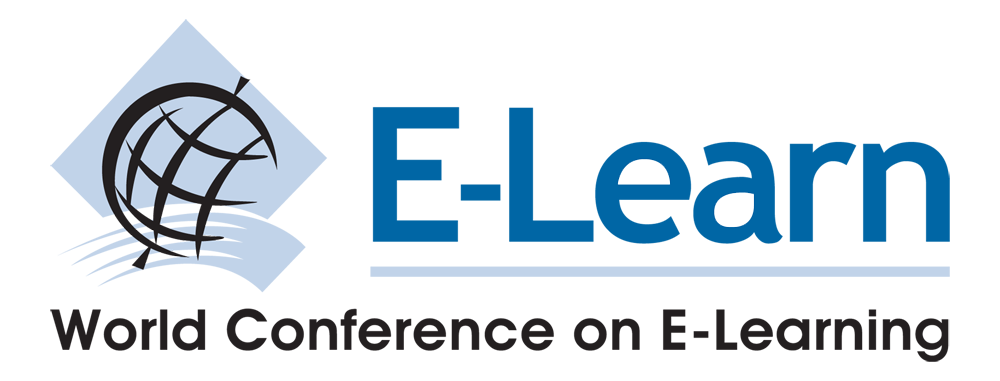
E-Learn: World Conference on E-Learning in Corporate, Government, Healthcare, and Higher Education, Oct 15, 2018 in Las Vegas, NV, United States ISBN 978-1-939797-35-3
Abstract
This paper study to predict whether students can graduate in four years at an early stage using machine learning. Four data sets are made from students' data containing Academic results and data before enrollment. Using these data sets, classification analysis by three machine learning algorithm and discrimination analysis were compared in Recall rate. It will be showed that it is useful to use data set containing academic results in the end of sophomore year and data before enrollment.
Citation
Hirose, H., Ozaki, T., Kawate, K., Yamamoto, Y. & Ichikawa, H. (2018). Construction of a Prediction Model of the Shortest Annual Graduation by Machine Learning Using Learning Environment Data. In Proceedings of E-Learn: World Conference on E-Learning in Corporate, Government, Healthcare, and Higher Education (pp. 510-515). Las Vegas, NV, United States: Association for the Advancement of Computing in Education (AACE). Retrieved August 9, 2024 from https://www.learntechlib.org/p/185001.
© 2018 AACE