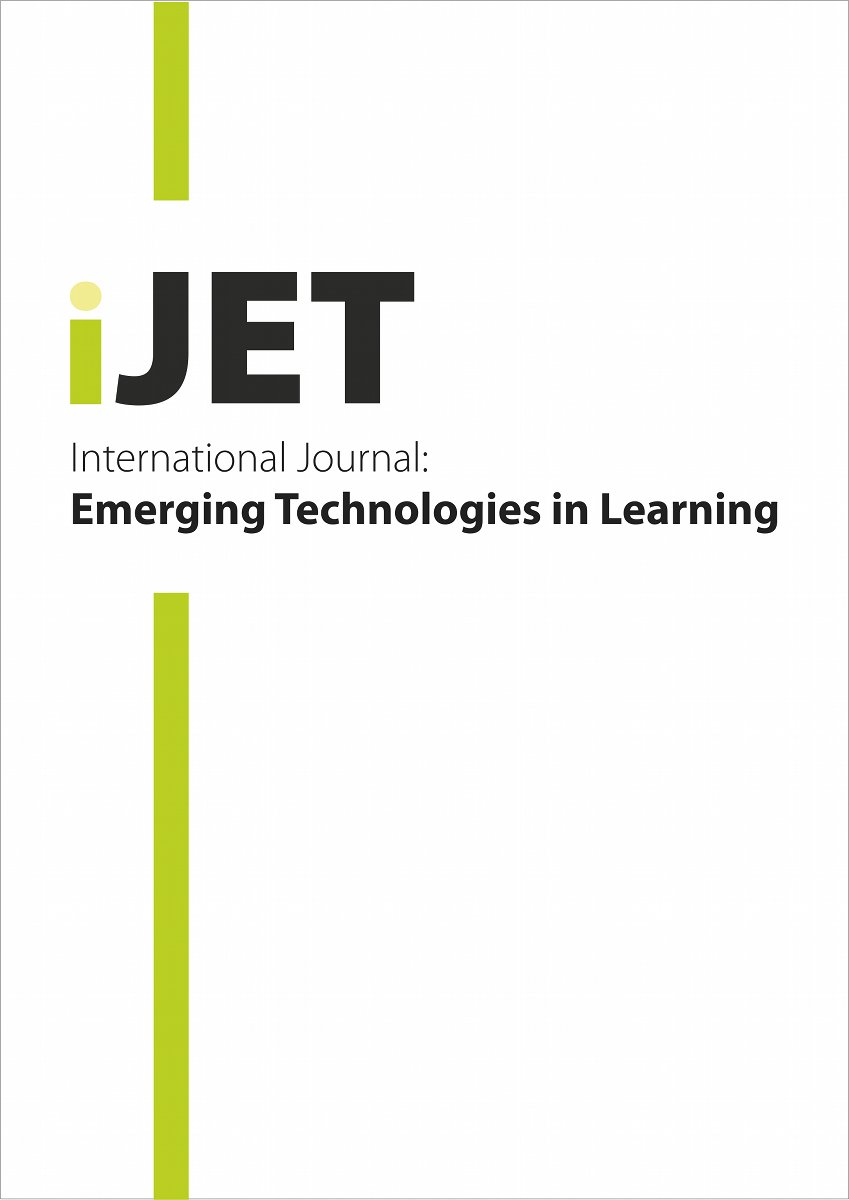
An Artificial Neural Network Based Early Prediction of Failure-Prone Students in Blended Learning Course
ARTICLE
Otgontsetseg Sukhbaatar, Tsuyoshi Usagawa, Kumamoto University ; Lodoiravsal Choimaa, National University of Mongolia
iJET Volume 14, Number 19, ISSN 1863-0383 Publisher: International Journal of Emerging Technology in Learning, Kassel, Germany
Abstract
One of the objectives of the performance measurement of grade-based higher education is to reduce the failure rate of students. To identify and reduce the number of failing students, the learning activities and behaviors of students in the classroom must be continuously monitored; however, monitoring a large number of students is an extremely difficult task. A penetration of web-based learning systems in academic institutions revealed the possibility of evaluating student activities via these systems. In this paper, we propose an early prediction scheme to identify students at risk of failing in a blended learning course. We employ a neural network on the set of prediction variables extracted from the online learning activities of students in a learning management system. The experiments were based on data from 1110 student who attended a compulsory, sophomore-level course. The results indicate that a neural-network-based approach can achieve early identification of students that are likely to fail; 25% of the failing students were correctly identified after the first quiz submission. After the mid-term examination, 65% of the failing students were correctly predicted.
Citation
Sukhbaatar, O., Usagawa, T. & Choimaa, L. (2019). An Artificial Neural Network Based Early Prediction of Failure-Prone Students in Blended Learning Course. International Journal of Emerging Technologies in Learning (iJET), 14(19), 77-92. Kassel, Germany: International Journal of Emerging Technology in Learning. Retrieved September 1, 2024 from https://www.learntechlib.org/p/217012/.