Example-Tracing Tutors: Intelligent Tutor Development for Non-Programmers
ARTICLE
Vincent Aleven, Bruce M. McLaren, Jonathan Sewall, Martin van Velsen, Octav Popescu, Sandra Demi, Michael Ringenberg, Kenneth R. Koedinger
IJAIE Volume 26, Number 1, ISSN 1560-4292
Abstract
In 2009, we reported on a new Intelligent Tutoring Systems (ITS) technology, example-tracing tutors, that can be built without programming using the Cognitive Tutor Authoring Tools (CTAT). Creating example-tracing tutors was shown to be 4-8 times as cost-effective as estimates for ITS development from the literature. Since 2009, CTAT and its associated learning management system, the "Tutorshop," have been extended and have been used for both research and real-world instruction. As evidence that example-tracing tutors are an effective and mature ITS paradigm, CTAT-built tutors have been used by approximately 44,000 students and account for 40% of the data sets in "DataShop," a large open repository for educational technology data sets. We review 18 example-tracing tutors built since 2009, which have been shown to be effective in helping students learn in real educational settings, often with large pre/post effect sizes. These tutors support a variety of pedagogical approaches, beyond step-based problem solving, including collaborative learning, educational games, and guided invention activities. CTAT and other ITS authoring tools illustrate that non-programmer approaches to building ITS are viable and useful and will likely play a key role in making ITS widespread.
Citation
Aleven, V., McLaren, B.M., Sewall, J., van Velsen, M., Popescu, O., Demi, S., Ringenberg, M. & Koedinger, K.R. (2016). Example-Tracing Tutors: Intelligent Tutor Development for Non-Programmers. International Journal of Artificial Intelligence in Education, 26(1), 224-269. Retrieved August 15, 2024 from https://www.learntechlib.org/p/176008/.
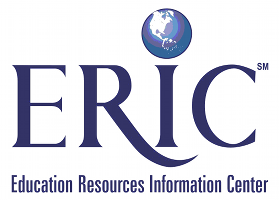
ERIC is sponsored by the Institute of Education Sciences (IES) of the U.S. Department of Education.
Copyright for this record is held by the content creator. For more details see ERIC's copyright policy.
Keywords
Cited By
View References & Citations Map-
More confusion and frustration, better learning: The impact of erroneous examples
J. Elizabeth Richey, Carnegie Mellon University, United States; Juan Miguel L. Andres-Bray, University of Pennsylvania, United States; Michael Mogessie, Carnegie Mellon University, United States; Richard Scruggs & Juliana M.A.L. Andres, University of Pennsylvania, United States; Jon R. Star, Harvard University, United States; Ryan S. Baker, University of Pennsylvania, United States; Bruce M. McLaren, Carnegie Mellon University, United States
Computers & Education Vol. 139, No. 1 (October 2019) pp. 173–190
These links are based on references which have been extracted automatically and may have some errors. If you see a mistake, please contact info@learntechlib.org.